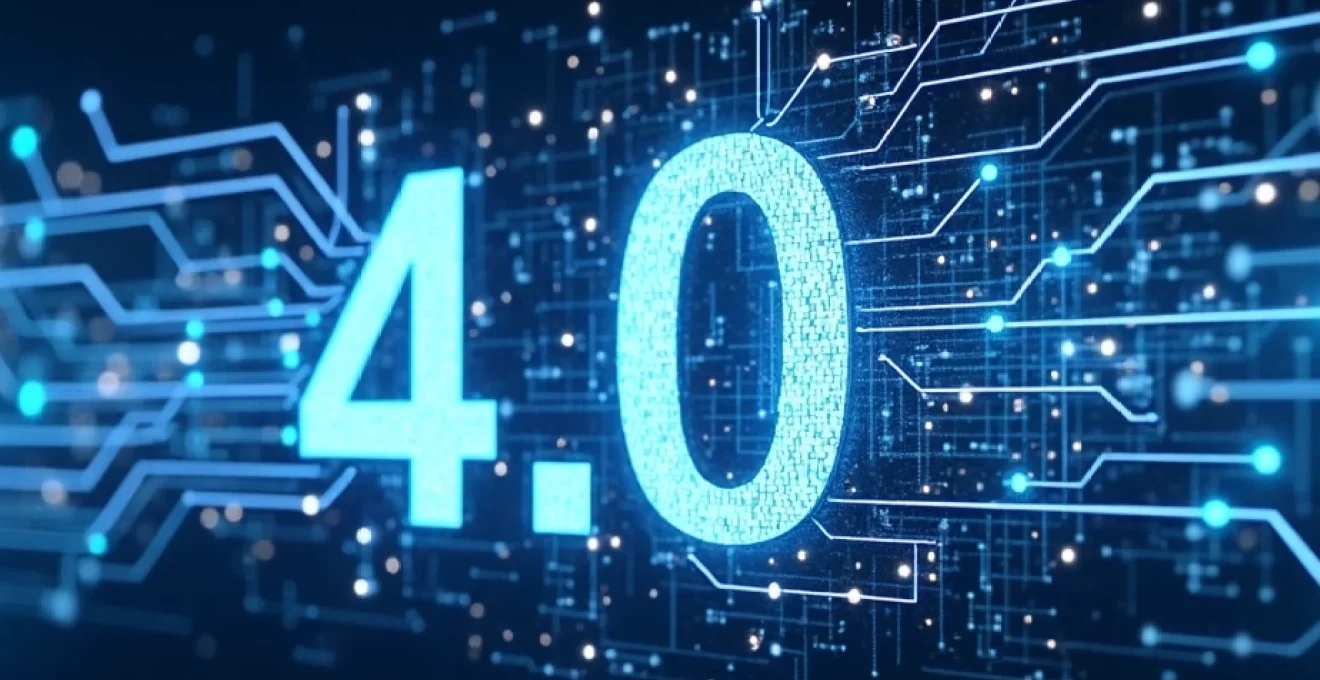
The fourth industrial revolution, known as Industry 4.0, is ushering in a new era of manufacturing and production. This digital transformation is reshaping traditional industries, bringing unprecedented levels of automation, connectivity, and data-driven decision-making to the factory floor. As technologies like artificial intelligence, the Internet of Things, and advanced robotics converge, businesses are reimagining their operations to stay competitive in an increasingly digital world.
Evolution of industry 4.0: from CPS to AI-driven manufacturing
Industry 4.0 has its roots in the concept of Cyber-Physical Systems (CPS), which integrate computational and physical processes. Over time, this foundation has evolved into a more comprehensive digital ecosystem. Today, AI-driven manufacturing represents the cutting edge of Industry 4.0, where machine learning algorithms optimize production processes in real-time.
The journey from CPS to AI-driven manufacturing has been marked by several key milestones. Initially, the focus was on connecting machines and gathering data. As technologies matured, the emphasis shifted to analyzing this data for insights. Now, we're entering a phase where AI can make autonomous decisions, predict maintenance needs, and even design new products.
This evolution has not been without challenges. Many companies have struggled with the complexity of integrating new technologies into legacy systems. Others have faced resistance from employees concerned about job security. However, those who have successfully navigated these obstacles are reaping significant rewards in terms of efficiency, productivity, and innovation.
Key technologies driving industry 4.0 transformation
Several cutting-edge technologies are at the heart of the Industry 4.0 revolution. These innovations are transforming manufacturing processes, supply chains, and business models across various sectors. Let's explore some of the most impactful technologies driving this digital transformation.
Industrial Internet of Things (IIoT) and smart sensors
The Industrial Internet of Things (IIoT) is a network of interconnected devices and sensors that collect and exchange data in industrial settings. Smart sensors are a crucial component of IIoT, providing real-time data on everything from temperature and pressure to vibration and energy consumption.
These technologies enable manufacturers to monitor their operations with unprecedented granularity. For example, a smart sensor on a production line can detect minute changes in equipment performance, allowing for predictive maintenance and reducing costly downtime. The data collected by IIoT devices also facilitates process optimization and quality control.
As the number of connected devices grows, so does the potential for innovation. Companies are finding new ways to leverage IIoT data to improve efficiency, reduce waste, and create new value-added services for their customers.
Big data analytics and cloud computing in manufacturing
The vast amounts of data generated by IIoT devices would be overwhelming without the power of big data analytics and cloud computing. These technologies allow manufacturers to process and analyze massive datasets, extracting valuable insights that can drive decision-making and improve operations.
Cloud computing provides the scalable infrastructure needed to store and process this data, while big data analytics tools help make sense of it all. For instance, a manufacturer might use these technologies to analyze production data across multiple facilities, identifying bottlenecks and optimizing resource allocation in real-time.
The combination of big data and cloud computing is also enabling new business models. Some companies are offering data-as-a-service to their customers, providing valuable insights derived from aggregated manufacturing data.
Artificial Intelligence and machine learning for predictive maintenance
Artificial Intelligence (AI) and Machine Learning (ML) are revolutionizing maintenance practices in manufacturing. By analyzing historical data and real-time sensor readings, AI algorithms can predict when equipment is likely to fail, allowing for proactive maintenance.
This predictive maintenance approach offers several benefits:
- Reduced downtime and maintenance costs
- Extended equipment lifespan
- Improved safety by preventing equipment failures
- Optimized inventory management for spare parts
Beyond maintenance, AI and ML are also being applied to quality control, process optimization, and even product design. As these technologies continue to advance, we can expect to see even more innovative applications in manufacturing.
Digital twin technology and simulation modeling
Digital twin technology creates a virtual replica of physical assets, processes, or systems. This digital representation can be used for simulation, analysis, and optimization without the need for physical prototypes or real-world testing.
In manufacturing, digital twins are used to:
- Simulate production processes to identify inefficiencies
- Test different scenarios without disrupting actual operations
- Train employees on new equipment or procedures in a safe, virtual environment
- Monitor and optimize asset performance in real-time
The power of digital twins lies in their ability to integrate real-time data from physical assets with historical data and advanced analytics. This combination allows for unprecedented insights and predictive capabilities.
Additive manufacturing and 3D printing advancements
Additive manufacturing, commonly known as 3D printing, is transforming product development and production processes. This technology allows for the creation of complex geometries that were previously impossible or prohibitively expensive to manufacture using traditional methods.
The benefits of additive manufacturing in Industry 4.0 include:
- Rapid prototyping and reduced time-to-market
- On-demand production of spare parts, reducing inventory costs
- Customization and mass personalization of products
- Reduction in material waste compared to subtractive manufacturing methods
As 3D printing technologies continue to advance, we're seeing new materials being used and larger, more complex parts being produced. This is opening up new possibilities in industries ranging from aerospace to healthcare.
Cybersecurity challenges in connected industrial ecosystems
As industrial systems become more connected and reliant on digital technologies, cybersecurity has emerged as a critical concern. The potential consequences of a cyber attack on an industrial facility can be severe, ranging from production disruptions to environmental disasters.
Some of the key cybersecurity challenges in Industry 4.0 include:
- Protecting legacy systems that were not designed with cybersecurity in mind
- Securing the vast number of IoT devices and sensors in industrial environments
- Ensuring data integrity and confidentiality across complex supply chains
- Defending against increasingly sophisticated cyber threats, including state-sponsored attacks
To address these challenges, manufacturers are adopting a range of cybersecurity measures. These include implementing robust authentication protocols, encrypting data in transit and at rest, and using AI-powered threat detection systems. Many companies are also adopting a zero trust security model, which assumes that no user or device should be trusted by default, even if they're already inside the network perimeter.
"In the age of Industry 4.0, cybersecurity is not just an IT issue—it's a fundamental business risk that needs to be managed at the highest levels of the organization."
As the industrial landscape continues to evolve, so too must cybersecurity strategies. Companies that prioritize cybersecurity as part of their digital transformation efforts will be better positioned to reap the benefits of Industry 4.0 while mitigating its risks.
Impact of industry 4.0 on supply chain management
Industry 4.0 is not just transforming manufacturing processes; it's also revolutionizing supply chain management. The integration of digital technologies is enabling more transparent, efficient, and resilient supply chains.
One of the most significant impacts is the increased visibility across the entire supply chain. IoT sensors and blockchain technology are making it possible to track products from raw materials to end consumers with unprecedented detail. This transparency helps companies identify bottlenecks, reduce waste, and respond more quickly to disruptions.
AI and machine learning are also playing a crucial role in supply chain optimization. These technologies can analyze vast amounts of data to forecast demand, optimize inventory levels, and even predict potential disruptions. For example, an AI system might analyze weather patterns, social media trends, and historical sales data to predict demand for a particular product in a specific region.
"The supply chain of the future will be a seamless, data-driven ecosystem that can anticipate and respond to changes in real-time."
Another important development is the concept of the digital supply network . Unlike traditional linear supply chains, digital supply networks are interconnected, intelligent systems that can dynamically adjust to changing conditions. This flexibility allows companies to respond more effectively to disruptions and changing customer demands.
As supply chains become more digitized, new challenges emerge. Cybersecurity is a major concern, as a breach anywhere in the supply chain could have far-reaching consequences. Additionally, companies need to develop new skills and capabilities to manage these complex, data-driven systems effectively.
Workforce transformation: upskilling for the digital industrial era
The transition to Industry 4.0 is not just about technology—it's also about people. As manufacturing becomes more digitized and automated, the skills required of workers are changing dramatically. This shift is creating both challenges and opportunities for the industrial workforce.
One of the most pressing issues is the need for upskilling and reskilling. Many traditional manufacturing jobs are being transformed or replaced by roles that require digital skills. For example, a machine operator may need to learn how to interact with digital interfaces and interpret data from sensors.
To address this skills gap, many companies are investing heavily in training and development programs. These initiatives often focus on areas such as:
- Data analysis and interpretation
- Programming and software development
- Cybersecurity awareness
- Digital tool proficiency
- Problem-solving and critical thinking in digital environments
In addition to technical skills, there's an increasing emphasis on soft skills such as adaptability, creativity, and collaboration. As routine tasks are automated, human workers are being called upon to handle more complex, non-routine challenges that require these uniquely human capabilities.
The rise of Industry 4.0 is also creating entirely new job categories. Roles such as IoT architect
, data scientist
, and robotics coordinator
are becoming increasingly common in manufacturing environments. These positions require a blend of technical knowledge and industry-specific expertise.
While the transition to Industry 4.0 may be disruptive for some workers, it also presents opportunities for career growth and development. Workers who embrace lifelong learning and adapt to the changing technological landscape will be well-positioned to thrive in the digital industrial era.
As you navigate this transformation, remember that the goal is not to replace human workers with machines, but to augment human capabilities with technology. The most successful Industry 4.0 implementations are those that find the right balance between human skills and technological capabilities.